Demography beyond a dissertation: profiting from well replicated and long term data
by Rob Salguero-Gomez on Apr 5, 2018
Although there are thousands of studies of population dynamics (Crone et al. 2011, Merow et al. 2014), most occur at small spatial scales and span few years (Menges 2000, Crone et al. 2011, Salguero-Gómez et al. 2015). We are persuaded that limited spatial replication and short study intervals can hinder our ability to adequately understand and predict population dynamics. In a recent publication (
Quintana-Ascencio et al. accepted) we used an unusually detailed, spatially expansive, long-term dataset to unravel complex interactions between landscape patterns and ecological disturbances affecting a species’ distribution and demography. We assessed the effects of landscape factors on the population dynamics of
Hypericum cumulicola, a pyrogenic, endangered Florida scrub endemic plant species. We took advantage of an ongoing 22-year study, that has monitored many populations (sampled in 15 independent habitat patches) with a rich disturbance history (14 independent fires), well-described habitat requirements (for open gaps in Florida scrub dominated by
Ceratiola ericoides) and a strong spatially patterned landscape.
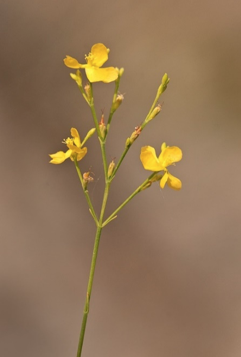
We use of a long-term dataset to decouple effects of disturbance from population and year effects. The effects of disturbance regimes on population dynamics can be challenging to study because of the large amount of longitudinal data required. Long-term studies may be necessary to avoid misleading conclusions built on chronosequence-based short-term studies. Even when such long-term data are available, decoupling the effects of time since disturbance from year and population effects (e.g. climate, biotic interactions) requires datasets with replication across space and time.
We have shown before that
H. cumulicola vital rates were strongly related to fire, the predominant ecological disturbance. Yet, in the reference study, we provide further evidence that vital rates were also affected by small scale landscape patterns such as elevation that alter the distance to the water table and larger scale patterns of patches in the landscape such as patch aggregation (e.g. survival was higher in large aggregated patches).
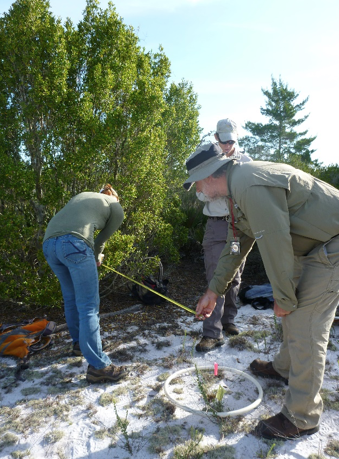
Overall, our results indicated the importance of metapopulation dynamics.
H. cumulicola occupancy and population growth predictions were highest in large, aggregated habitat patches. Large habitat patches may have several advantages for metapopulation dynamics, including a larger target for rescues, greater diversity of microhabitats, and the ability to support larger populations less prone to demographic or environmental stochasticity (Hanski, Moilanen & Gyllenberg 1996). Larger habitat patches may also be more likely to support larger populations of the dominant shrub Florida rosemary, which may act as a foundation species for
H. cumulicola and other herbaceous plants that are vulnerable to competition from resprouting shrubs. Model predictions of abundance were most reliable for patches with the extremes of patch area or aggregation. In this study, predictions of occupancy and abundance were frailer for habitat patches with small and intermediate size and aggregation. We have previously documented that unoccupied patches can be suitable habitat for
H. cumulicola. These results indicate that limited dispersal and unfavorable matrix habitats can synergistically contribute to colonization failure. Few studies have integrated population models and landscape level environmental drivers to characterize species distributions, although this approach has great promise for assessing the consequences of environmental changes
The distribution of species across landscapes ultimately reflects the interaction of demography with landscape and disturbance properties. However, both demographic inertia (e.g., long life span, dormant stages) and landscape history (e.g. environmental legacies) may create lags in responses. Therefore, realized species distributions (reflecting past interactions) may have different patterns than current vital rates. We used this detailed long-term monitoring to assess the relationships between demography and landscape-level drivers to disentangle the potential causes of realized species distributions.
H. cumulicola occupancy peaked at higher elevations in larger patches but many vital rates peaked at lower elevations. This may reflect lags in demography such as the role of seed banks in allowing populations to persist between disturbances. Better understanding of the spatial and temporal dynamics of seed dormancy and dispersal and the role of environmental factors on their variation will greatly benefit our understanding of regional plant population persistence. In addition, landscape changes in extreme microsites (e.g. the largest open patches) may lag behind other landscape patterns. Short-term or limited studies in areas with these persisting patches may be biased, as patches where all plants have died cannot be a source of data. This demographic ghost of mortality past may explain unexpected demographic patterns in chronosequence studies.
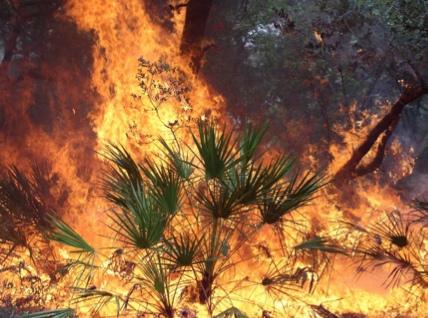
Many critiques of demographic modeling have pointed out that models do a poor job of predicting beyond more than a few years (Crone 2013). By incorporating common drivers such as weather, habitat suitability, disturbances, landscape patterns, and land management, we can improve our understanding of temporal and spatial variability in demography (Ehrlén & Morris 2015). Weaknesses of population models can be attributed to their frequent dependence on short spans of data (Menges 2000) and on their frequent blindness to underlying drivers (Ehrlén & Morris 2015). The scaling up of local demography to landscapes, accomplished by a combination of approaches, can lead to more effective management for ecological diversity.
Pedro F Quintana Ascencio and
Eric S Menges
References
Crone, E.E., et al. (2011) How do plant ecologist use matrix population models?
Ecology Letters, 14, 1-8.
Ehrlén, J. & Morris, W.F. (2015) Prediction changes in the distribution and abundance of species under environmental change.
Ecology Letters, 18, 303-314.
Hanski, I., Moilanen, A. & Gyllenberg, M. (1996) Minimum viable metapopulation size.
American Naturalist, 147, 527-541.
Menges, E.S. (2000) Population viability analyses in plants: challenges and opportunities.
Trends in Ecology and Evolution 15, 51-56.
Merow, C.
et al. (2014) Advancing population ecology with integral projection models: a practical guide.
Methods in Ecology and Evolution 5, 99-110.
Quintana-Ascencio, P.F., Koontz, S., Smith, S. Sclater, V. David, A., Menges, E. S. (2018) Predicting landscape-level distribution and abundance: Integrating demography, fire, elevation, and landscape habitat configuration.
Journal of Ecology, Accepted manuscript online: 2 APR 2018 12:00AM EST | DOI: 10.1111/1365-2745.12985
Salguero-Gómez,
et al. (2015) The COMPADRE plant matrix database: an open online repository for plant demography.
Journal of Ecology 103, 202-218.